
- Structured data generator how to#
- Structured data generator generator#
- Structured data generator code#
def data_generator(data,batch_size=10,shuffle=True): """ Yields the next training batch.
Structured data generator generator#
For instance, if temporal_length was 16 and the number of frames in the video is less than 16 (say even 15), we will drop this video.Īs discussed in part -2 of this tutorial series, the template which we will be following for creating a custom data generator is taken from this amazing blog - Now we have loaded our sample, we will feed this loaded sample into the data generator. The first sample will be from frame 1 to frame 16, the next will be from frame 5 to frame 21, the nest from frame 9 to 25, and so on.įor now, we will not consider temporal padding i.e if the number of frames in a video is less than the temporal_length we will drop that video from our data-set. If you choose a temporal stride of 4 then the number of data samples that we can get is 9. How many data samples can be prepared from this video? The first sample will be from frame 1 to frame 16, the next from frame 2 to frame 17, and the next from 3 to 18. Say you chose a temporal dimension of 16 and a temporal stride of 1. The next step is to write the paths and labels of each frame in a CSV file as shown in the figure below.
Structured data generator code#
The code to do this is pretty straightforward root_dir = ‘UCF-101’ # source dir dest_dir = ‘activity_data’ # destination directory # create the destination dir if it does not exists if not os.path.exists(dest_dir): os.mkdir(dest_dir) # To list what are the directories - train, test data_dir_list = os.listdir(root_dir) for data_dir in data_dir_list: # loop over train and test dir data_path = os.path.join(root_dir,data_dir) #Read all the folders in each of the train and test dir dest_data_path = os.path.join(dest_dir,data_dir) if not os.path.exists(dest_data_path): os.mkdir(dest_data_path) # for each of the activity - Archery, Basketball and Biking #read all the videos in the activity list activity_list = os.listdir(data_path) # for each of the video in video list, read the video and save #every frame for activity in activity_list: activity_path = os.path.join(data_path,activity) dest_activity_path = os.path.join(dest_data_path,activity) if not os.path.exists(dest_activity_path): os.mkdir(dest_activity_path) write_frames(activity_path,dest_activity_path)ģ. Īfter step the structure becomes activity_recognition UCF-101. This will be our working directory from hereīefore step 2 the structure was activity_recognition UCF-101 train Archery v_Archery_g01_c01.avi v_Archery_g01_c02.avi. we will create a new directory called ‘activity_data’ where all the videos will be saved as frames.
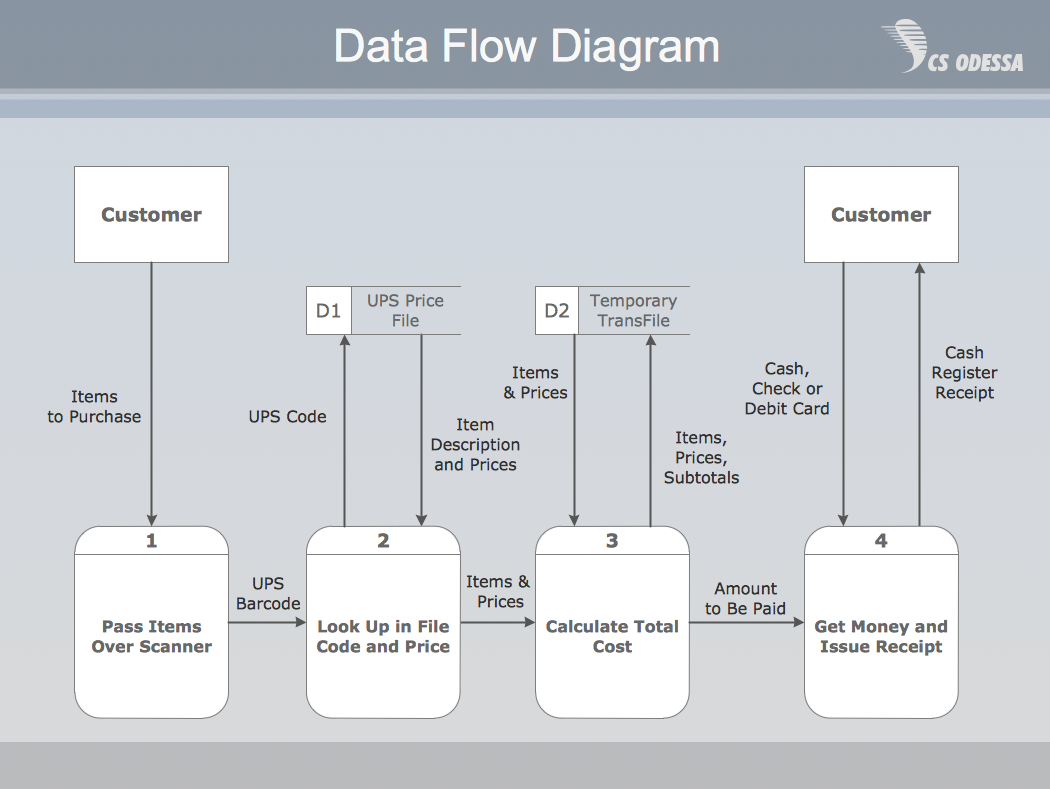
And video data sets are usually saved in this format. this will help us to load the data frame by frame.

In this post, we will write a data generator for the second and third scenarios.
Structured data generator how to#
In the previous post, we discussed how to write a data generator for the first scenario and if you haven’t been through the previous post, I would suggest that you go through that first - Chapter-2: Writing a generator function to read your data that can be fed for training an image classifier in Keras.
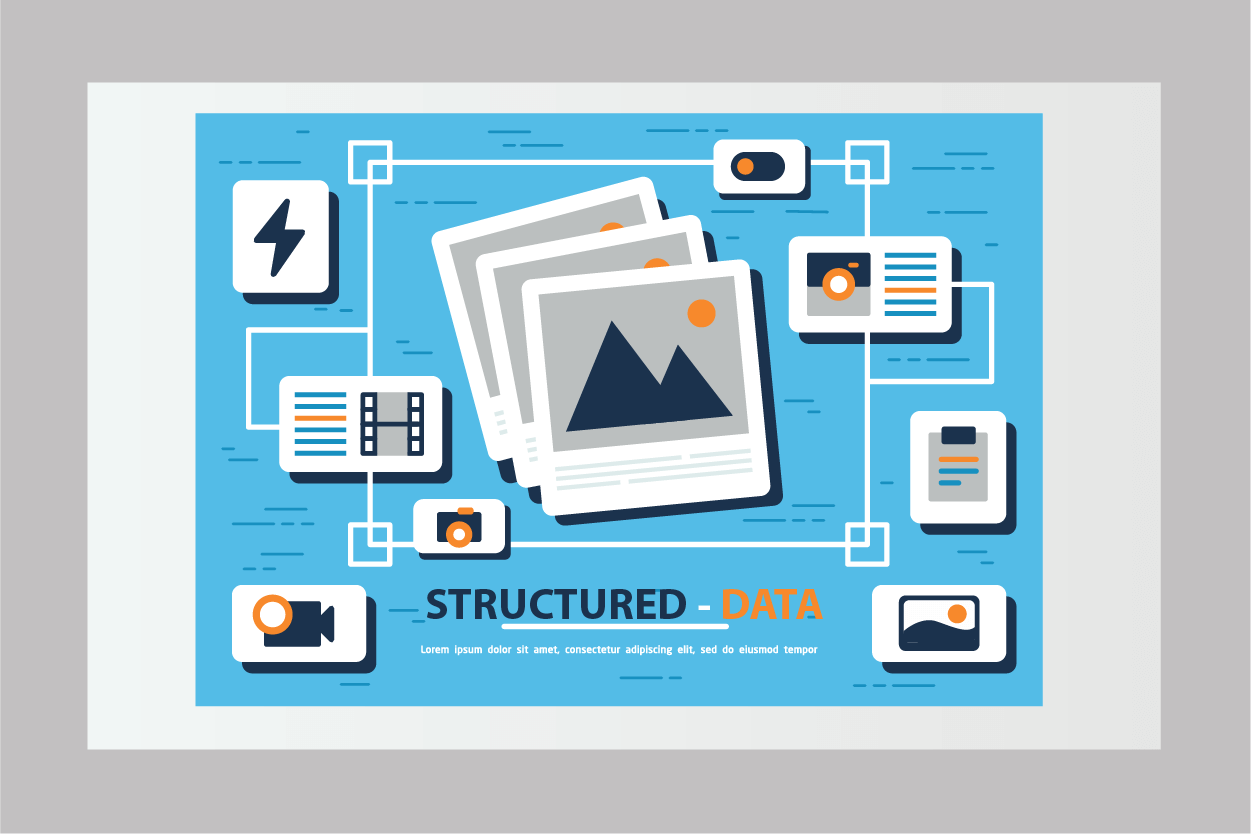
Scenario-3: When you have multiple inputs that need to be fed to an ensemble of model
